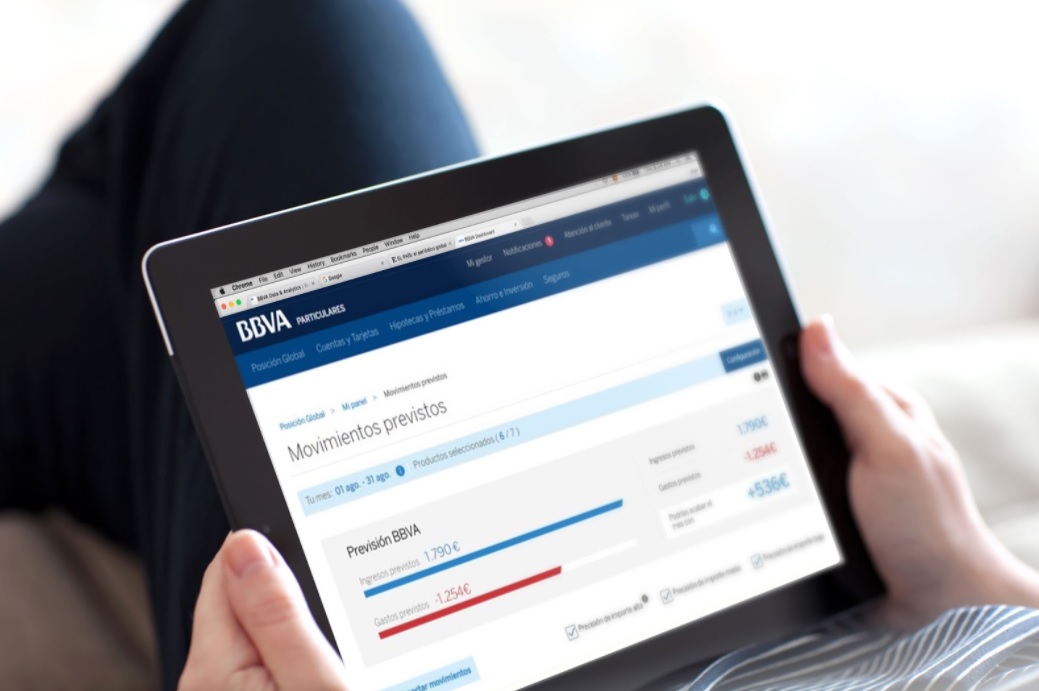
Improving Customer Experience with Forecasting Models
Direct debit and credit cards have made the lives of many easier. They have allowed us to save time and headaches, and helped us finance goods that exceed our monthly purchasing power. Sometimes, they have also created situations when an unforeseen payments came through in an unexpected moment with a low balance the missing payment involves a penalty.
To solve this issue BBVA has recently launched a Finance Calendar feature as part of its web and mobile apps. Called “Expense and Income Forecast”, it helps customers gain control over their account by providing estimations of future recurring income and expenses. This feature is now available as part BBVA’s Personal Finance Manager (PFM) called “My Day to Day”, which is currently used by 1 million clients each month.
The core algorithm, its forecasting engine, was developed by BBVA Data & Analytics using Big Data tools like Apache Spark and implementing a combination of Machine Learning algorithms for time series forecasting. It is another example of how BBVA Data & Analytics applies Data science to improve the financial life of BBVA clients by providing useful information to take decisions.
Customers can see two types of forecasts. On the one hand, the total amount of expenses and incomes expected in a set of financial categories (like groceries, education, or leisure) for the current month. On the other hand, for operations which present a strong temporal recurrent pattern, these are associated with a tentative data and displayed in a calendar. Therefore, customers get a picture of when in the future they will receive a payment or a direct debit from the electricity company or a mortgage, and can prepare for other extraordinary payments that may impact their account balance.
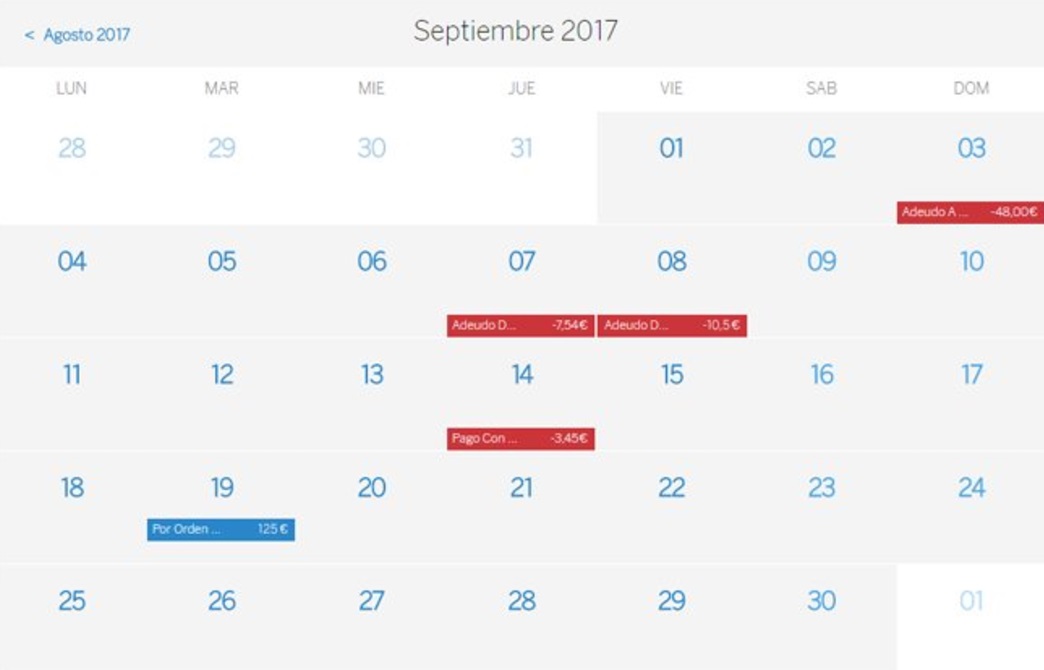
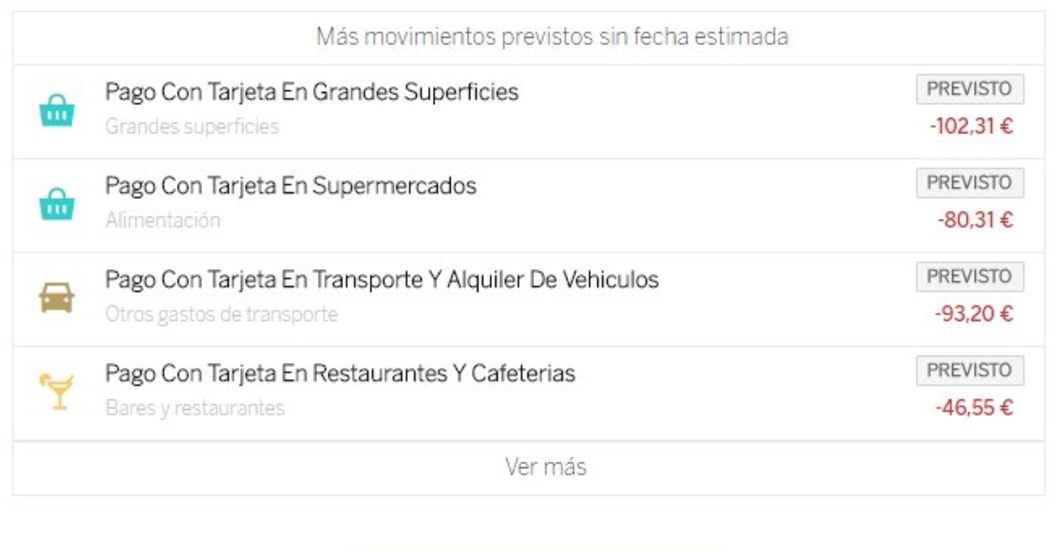
Data Sources and Analytical Framework
The new feature reads historical data of account transactions to identify recurrent income and payments categories and determine an approximate amount and date for the next 2 months, combining methods of Time Series analysis and an ensemble of Machine Learning models.
This model generates around 30 million predictions per month, with an accuracy of 85%. Predicted amounts will differ 12% or less with the real value for half of the cases. For each prediction the model provides a “Confidence tag” that allows clients to know which amount predictions are expected to be more precise than others.
The feature is the one step further in an continuous research process, some previous results of this research was showed in a poster entitled Evaluating uncertainty scores for deep regression networks in financial short time series forecasting that was presented in the Workshop on Machine Learning for Spatiotemporal Forecasting as part of NIPS 2016
Results
After some months in beta testing with BBVA’s employees, the engine is now open for all BBVA’s clients in Spain. This an example of Machine Learning in production stage, it is also an applied case Financial Technology (Fintech) for improving the experience between people and banks.