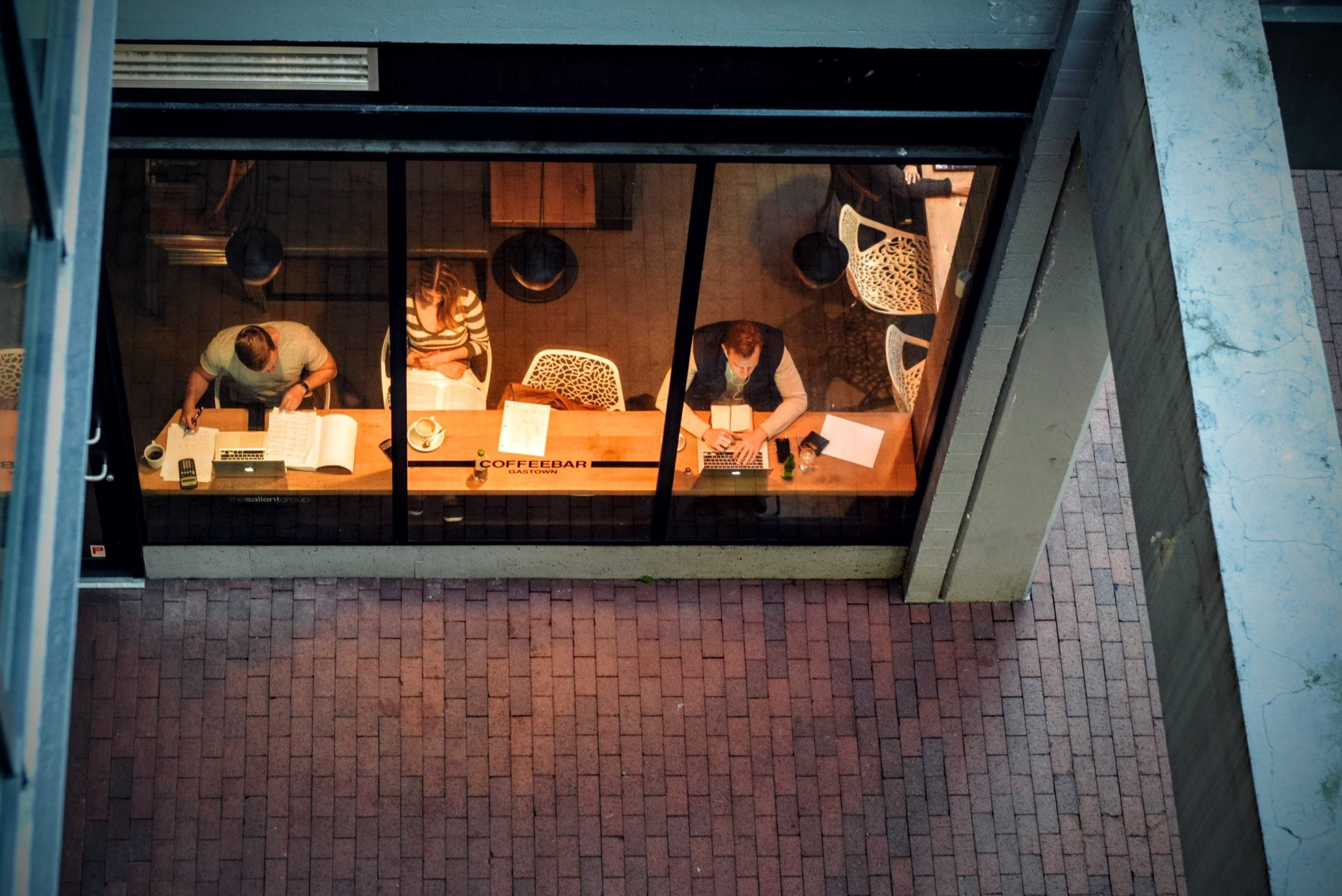
The #10yearchallenge of Data Science
Ten years ago the term “Data Science” was only 7% of what it is today in Google Trends. It was almost non-existent in the news, and only timidly gaining ground in the corporate narrative. One has to go back to 2010 to see a first comprehensive definition of the nascent discipline of data science in the media. The Economist ran a special report that refers to the new craftsmanship of a data scientist as the combination of the “skills of software programmer, statistician and storyteller/artist to extract the nuggets of gold hidden under mountains of data”.
The discipline of data science has skyrocketed in the last decade, turning economics, social science and business strategies upside-down. At the moment, the discipline of data science is the driver behind more than 250 Master’s degree programs in the US, according to a report by the Institute for Advanced Analytics (North Carolina State University), which in 2007 created the first-ever MSc in Analytics in the country.
Corporations are now craving for data scientists. The term “data scientist” went mainstream when DJ Patil introduced it to the world in his famous book “Data Jujitsu–The art of turning data into products”. Financial institutions went from hiring “quants” to headhunting “data scientists”, hoping they would apply their “magic” to the vast amounts of data lying around in order to launch new products, create efficiencies and extract new insights. The story was not that simple: several industry polls have recently shown frustration vis-à-vis businesses when applying data science to productive processes. One of the greatest challenges has been to find the right integration with other teams such as business development, design, engineering, or ethics.
The original definition of a data scientist (i.e., someone with a perfect blend of computing skills, math, and statistical knowledge, and with domain expertise to develop business cases) has greatly changed in the last few years shaped by reality. Corporations discovered, one way or the other, that the application of data science tools has to be paired with cultural transformation, design, agile development, foresight, and a proper formulation of business questions and hypothesis.
What DJ Patil eventually came to realize is that the job of a data scientist is “amorphous. There’s no specific thing that you do”, according to an interview published in 2016.
From 0 to 100 in 10 years
Machine Learning techniques such as today’s ubiquitous Deep Learning or Reinforcement Learning were barely known 10 years ago, and are now part of the jargon of corporations, governments, and technologists.
In 2009, the statistician Nate Silver, co-founder of FiveThirtyEight, was named one of the 100 most influential people by Time Magazine for building models to forecast baseball champions and presidential election outcomes. Suddenly, statistics was referred to as the “sexiest job” around. Statistical expertise and growing computational processing capabilities, plus cloud computing (Amazon Web Services was born just 3 years earlier), was the perfect breeding ground for of data science. The new discipline was instrumental in the renaissance of Artificial Intelligence (AI). Today, we use the word AI to name tasks that were non- existent or merely experimental only a decade ago, including voice assistants, translation, facial recognition, and self-driving cars.
Data Science and the democratization of knowledge
The fact that the boundaries of data science were not defined – even to this day-, and that data science didn’t provide the answer to some rigid academic traditions contributed to the development of very democratic and open discipline. Researchers and aspiring data scientists from all over the world would come together to test new analytical approaches together with new applications of techniques for speeding up mundane tasks, such as tagging data.
Great breakthroughs came about because people like Fei-Fei Li and her team had the vision and the drive to crowdsource the annotation of 14 million images. This endeavor was instrumental in the training of Deep Learning models and in enabling self-driving cars, among other applications. Places like Kaggle contribute to open datasets, share knowledge and techniques, and create a sense of community and competition where data science has been able to thrive.
However, one of the most impactful events for the democratization of data science was the launch in 2012 of the learning platform Coursera founded by Andrew Ng and Daphne Koller. Coursera has since helped train millions of data scientists, data engineers or data analysts with free and open courses taught by the best computer scientists, statisticians, and economists around the globe. All of a sudden, data science’s cutting-edge knowledge has become available to anyone for free.
Ten years of Data Science has brought breakthroughs such as facial recognition, autonomous cars, voice assistants, forecasting for financial services, urban planning or decision making. It has also elevated Artificial Intelligence to a set of tools that can be translated into every-day products and which is here to stay… at least until the singularity takes over.